17. 9 月 2018
Research Projects
VR in Automotive
A series of collaborations with VW and Audi which want to collaborate in VR. Work investigating high-end renderings, construction validation, virtual installations and ergonomics. We focused on analyzing latencies in distributed VR.
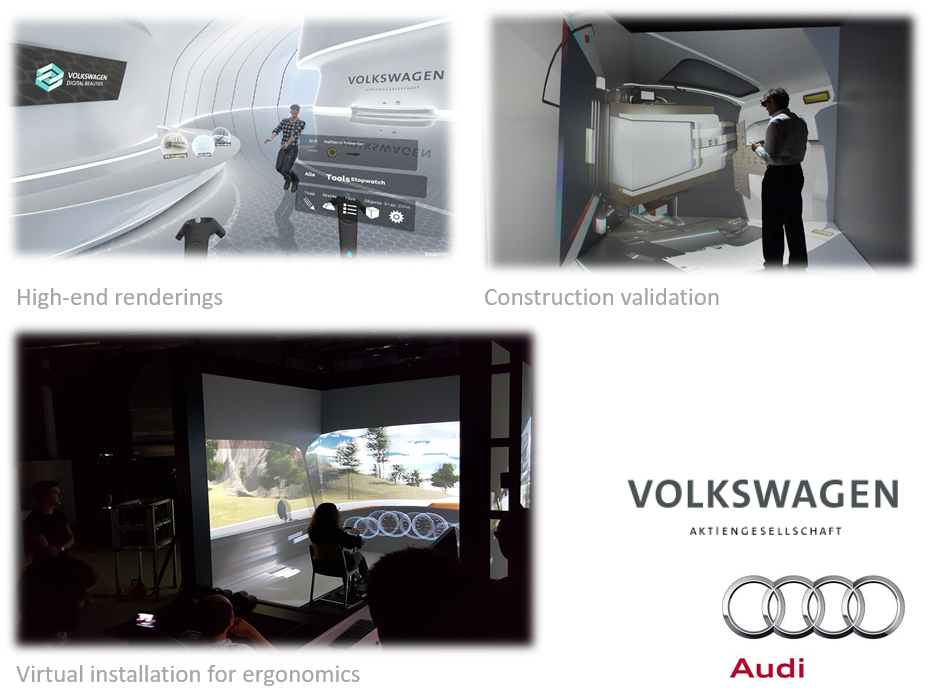
VR in Art and Historical Projects
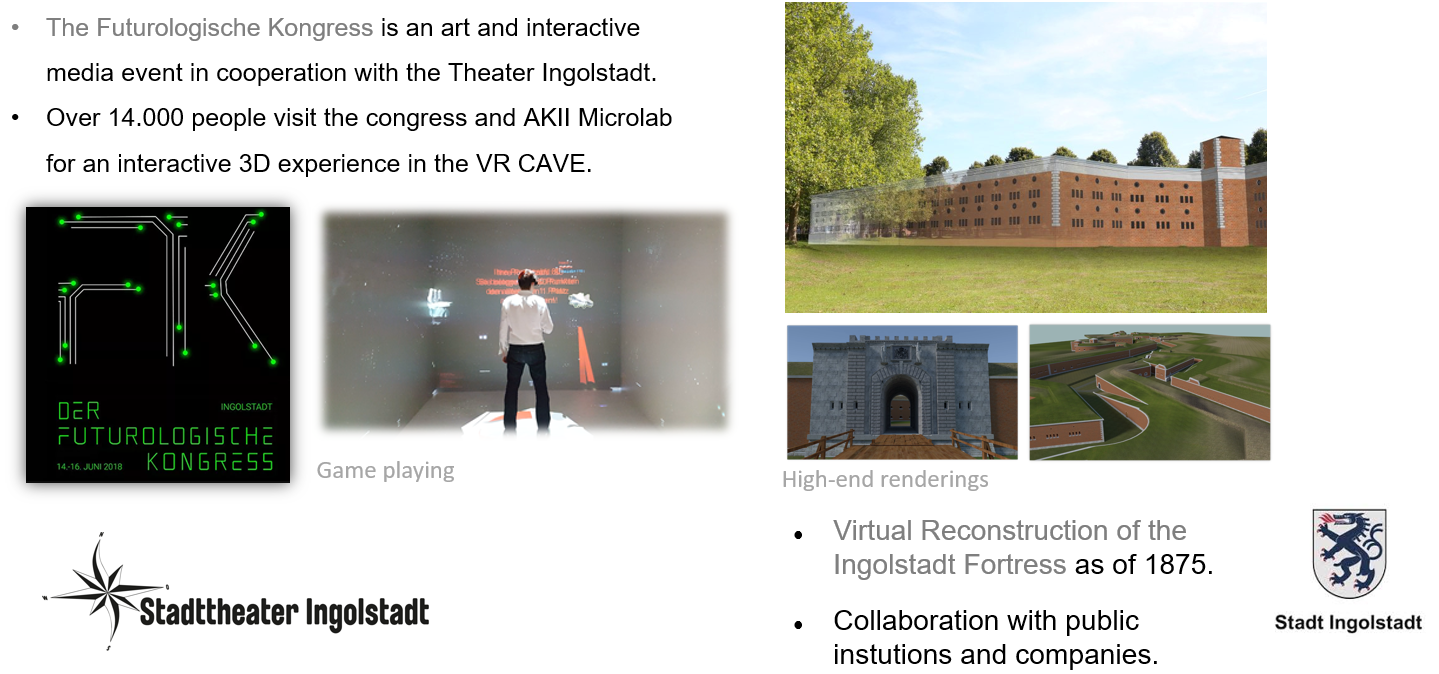
VR for Rehabilitation
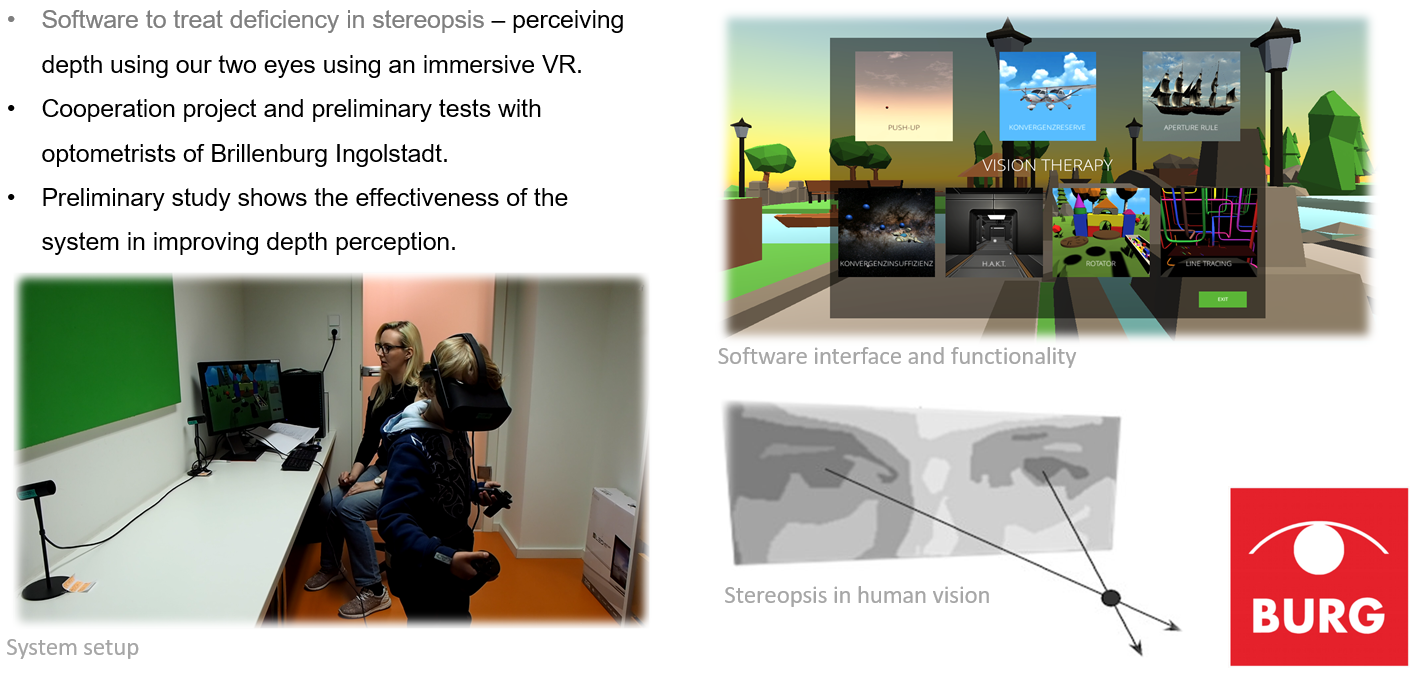
Adaptive Neuromorphic Sensorimotor Control
Efficient sensorimotor processing is inherently driven by physical real-world constraints that an acting agent faces in its environment. Sensory streams contain certain statistical dependencies determined by the structure of the world, which impose constraints on a system’s sensorimotor affordances.
This limits the number of possible sensory information patterns and plausible motor actions. Learning mechanisms allow the system to extract the underlying correlations in sensorimotor streams.
This research direction focused on the exploration of sensorimotor learning paradigms for embedding adaptive behaviors in robotic system and demonstrate flexible control systems using neuromorphic hardware and neural-based adaptive control. I employed large-scale neural networks for gathering and processing complex sensory information, learning sensorimotor contingencies, and providing adaptive responses.
To investigate the properties of such systems, I developed flexible embodied robot platforms and integrate them within a rich tool suite for specifying neural algorithms that can be implemented in embedded neuromorphic hardware.
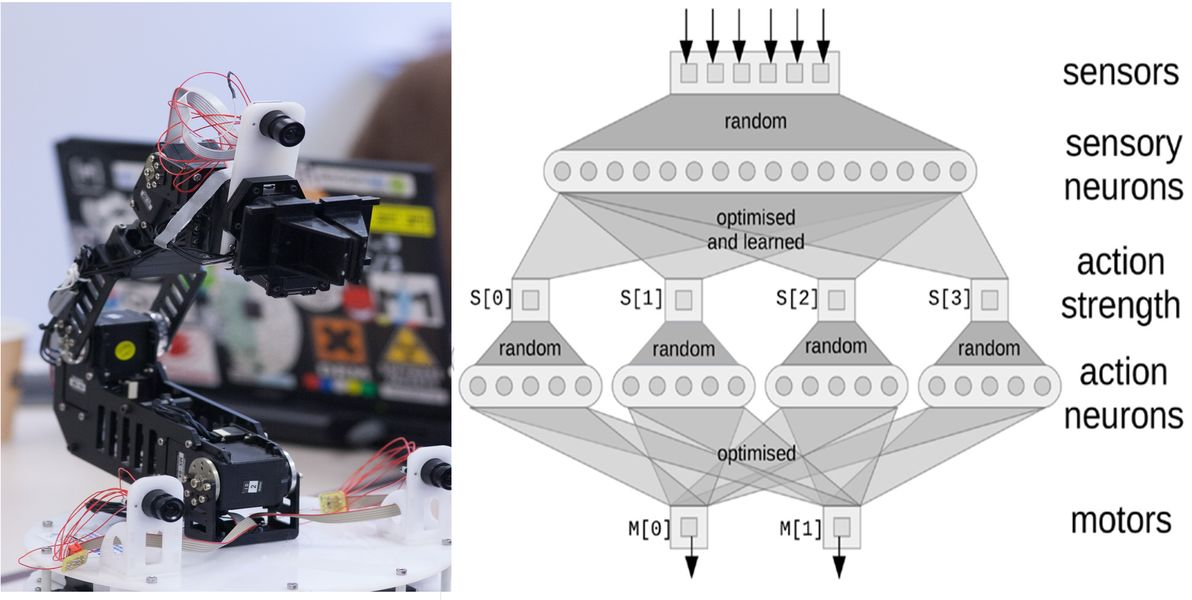
The mobile manipulator I developed at NST for adaptive sensorimotor systems consists of an omni-directional (holonomic) mobile manipulation platform with embedded low-level motor control and multimodal sensors.
The on-board micro-controller receives desired commands via WiFi and continuously adapts the platform’s velocity controller. The robot’s integrated sensors include wheel encoders for estimating odometry, a 9DoF inertial measurement unit, a proximity bump-sensor ring and three event-based embedded dynamic vision sensors (eDVS) for visual input.
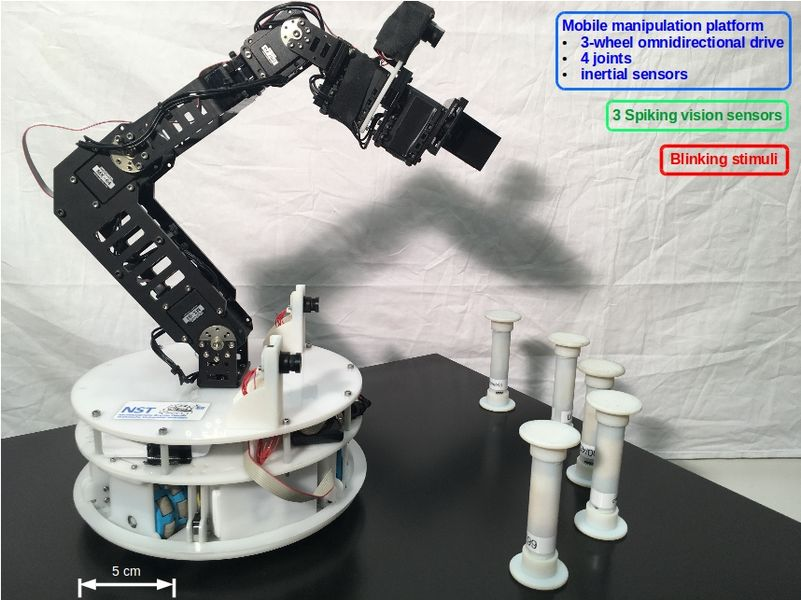
The mobile platform carries an optional 6 axis robotic arm with a reach of >40cm. This robotic arm is composed of a set of links connected together by revolute joints and allows lifting objects of up to 800 grams. The mobile platform contains an on-board battery of 360 Wh, which allows autonomous operation for well above 5h.
Synthesis of Distributed Cognitive Systems – Learning and Development of Multisensory Integration
My research interest is in developing sensor fusion mechanisms for robotic applications. In order to extend the framework of the interacting areas a second direction in my research focuses on learning and development mechanisms.
Human perception improves through exposure to the environment. A wealth of sensory streams which provide a rich experience continuously refine the internal representations of the environment and own state. Furthermore, these representations determine more precise motor planning.
An essential component in motor planning and navigation, in both real and artificial systems, is egomotion estimation. Given the multimodal nature of the sensory cues, learning crossmodal correlations improves the precision and flexibility of motion estimates.
During development, the biological nervous system must constantly combine various sources of information and moreover track and anticipate changes in one or more of the cues. Furthermore, the adaptive development of the functional organisation of the cortical areas seems to depend strongly on the available sensory inputs, which gradually sharpen their response, given the constraints imposed by the cross-sensory relations.
Learning processes which take place during the development of a biological nervous system enable it to extract mappings between external stimuli and its internal state. Precise ego-motion estimation is essential to keep these external and internal cues coherent given the rich multisensory environment. In this work we present a learning model which, given various sensory inputs, converges to a state providing a coherent representation of the sensory space and the cross-sensory relations.
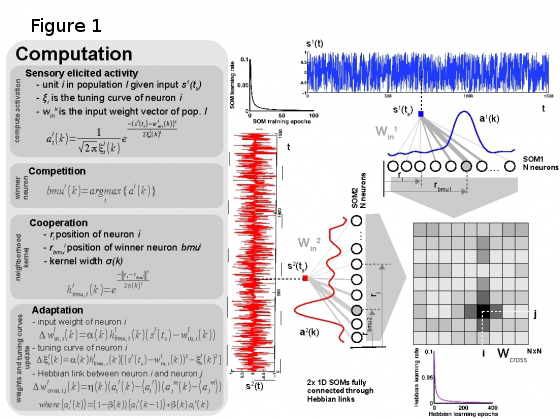
The model is based on Self-Organizing-Maps and Hebbian learning (see Figure 1) using sparse population coded representations of sensory data. The SOM is used to represent the sensory data, while the Hebbian linkage extracts the coactivation pattern given the input modalities eliciting peaks of activity in the neural populations. The model was able to learn the intrinsic sensory data statistics without any prior knowledge (see Figure 2).
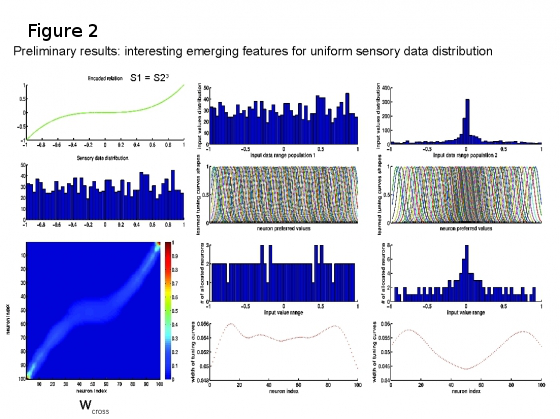
The developed model, implemented for 3D egomotion estimation on a quadrotor, provides precise estimates for roll, pitch and yaw angles (setup depicted in Figure 3a, b).
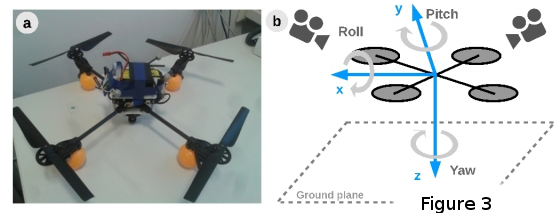
Given relatively complex and multimodal scenarios in which robotic systems operate, with noisy and partially observable environment features, the capability to precisely and timely extract estimates of egomotion critically influences the set of possible actions.
Utilizing simple and computationally effective mechanisms, the proposed model is able to learn the intrinsic correlational structure of sensory data and provide more precise estimates of egomotion (see Figure 4a, b).
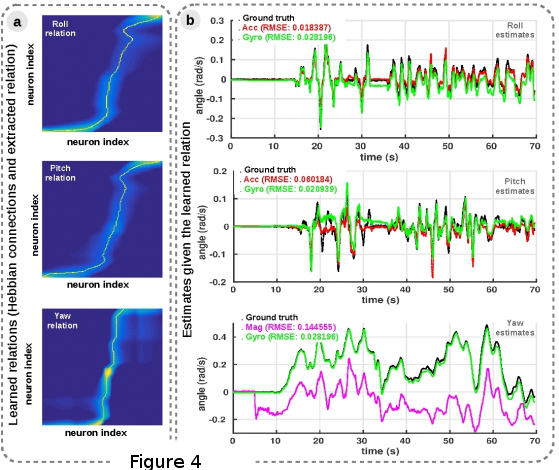
Moreover, by learning the sensory data statistics and distribution, the model is able to judiciously allocate resources for efficient representation and computation without any prior assumptions and simplifications. Alleviating the need for tedious design and parametrisation, it provides a flexible and robust approach to multisensory fusion, making it a promising candidate for robotic applications.
Synthesis of Distributed Cognitive Systems – Interacting Cortical Maps for Environmental Interpretation
The core focus of my research interest is in developing sensor fusion mechanisms for robotic applications. These mechanisms enable a robot to obtain a consistent and global percept of its environment using available sensors by learning correlations between them in a distributed processing scheme inspired by cortical mechanisms.
Environmental interaction is a significant aspect in the life of every physical entity, which allows the updating of its internal state and acquiring new behaviors. Such interaction is performed by repeated iterations of a perception-cognition-action cycle, in which the entity acquires and memorizes relevant information from the noisily and partially observable environment, to develop a set of applicable behaviors (see Figure 5).
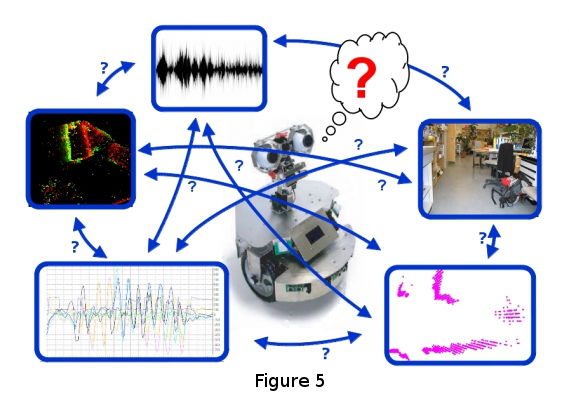
This recently started research project is in the area of mobile robotics, and more specifically in explicit methods applicable for acquiring and maintaining such environmental representations. State-of-the-art implementations build upon probabilistic reasoning algorithms, which typically aim at optimal solutions with the cost of high processing requirements.
In this project, we have developed an alternative, neurobiologically inspired method for real-time interpretation of sensory stimuli in mobile robotic systems: a distributed networked system with inter-merged information storage and processing that allows efficient parallel reasoning. This networked architecture will be comprised of interconnected heterogeneous software units, each encoding a different feature about the state of the environment that is represented by a local representation (see Figure 6).
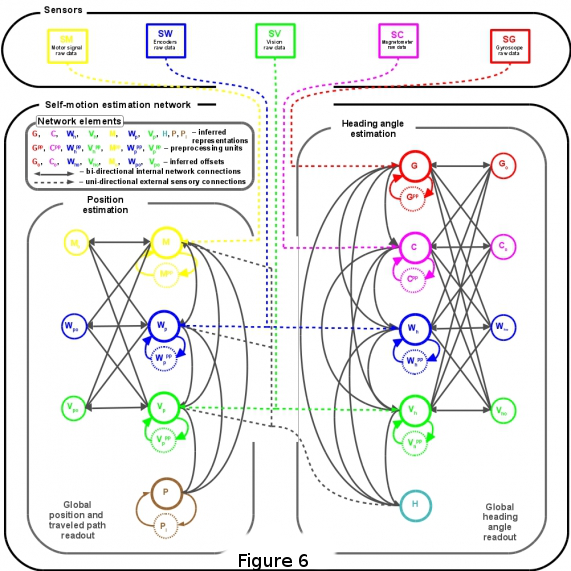
Such extracted pieces of environmental knowledge interact by mutual influence to ensure overall system coherence. A sample instantiation of the developed system focuses on mobile robot heading estimation (see Figure 7). In order to obtain a robust and unambiguous description of robot’s current orientation within its environment inertial, proprioceptive and visual cues are fused (see image). Given available sensory data, the network relaxes to a globally consistent estimate of the robot’s heading angle and position.
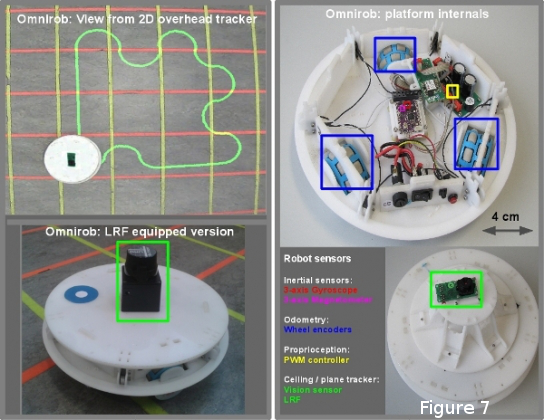
Adaptive Nonlinear Control Algorithm for Fault-Tolerant Robot Navigation
Today’s trends in control engineering and robotics are blending gradually into a slightly challenging area, the development of fault-tolerant real-time applications. Hence, applications should timely deliver synchronized data-sets, minimize latency in their response and meet their performance specifications in the presence of disturbances. The fault-tolerant behavior in mobile robots refers to the possibility to autonomously detect and identify faults as well as the capability to continue operating after a fault occurred. This work introduces a real-time distributed control application with fault tolerance capabilities for differential wheeled mobile robots (see Figure 8).
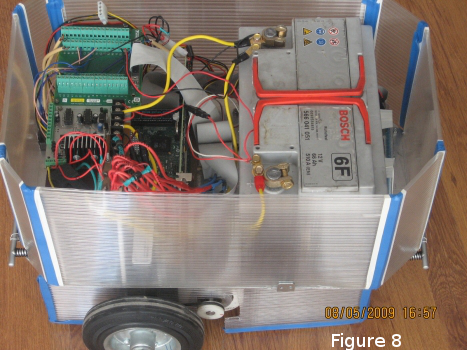
You are currently viewing a placeholder content from YouTube. To access the actual content, click the button below. Please note that doing so will share data with third-party providers.
More Information
Furthermore, the application was extended to introduce a novel implementation for limited sensor mobile robots environment mapping. The developed algorithm is a SLAM implementation. It uses real-time data acquired from the sonar ring and uses this information to feed the mapping module for offline mapping (see Figure 9).
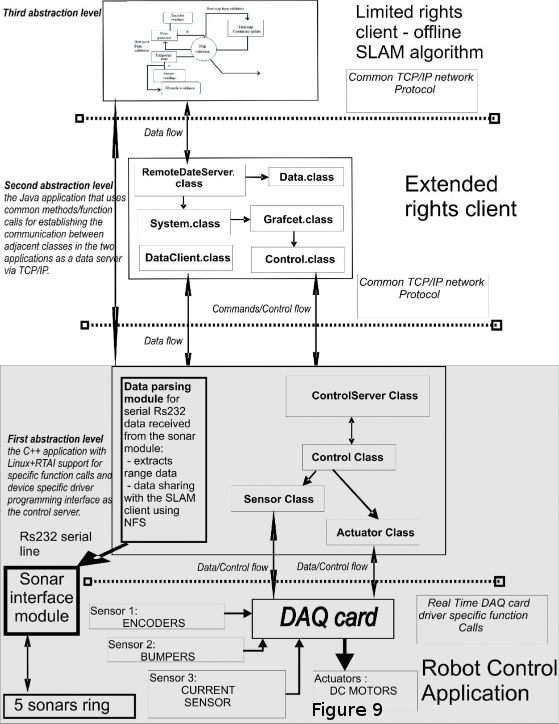
The latter is running on top of the real-time fault-tolerant control application for mobile robot trajectory tracking operation (see Figures 10, 11).
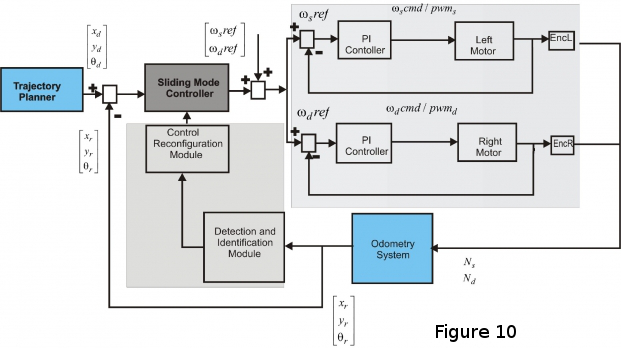
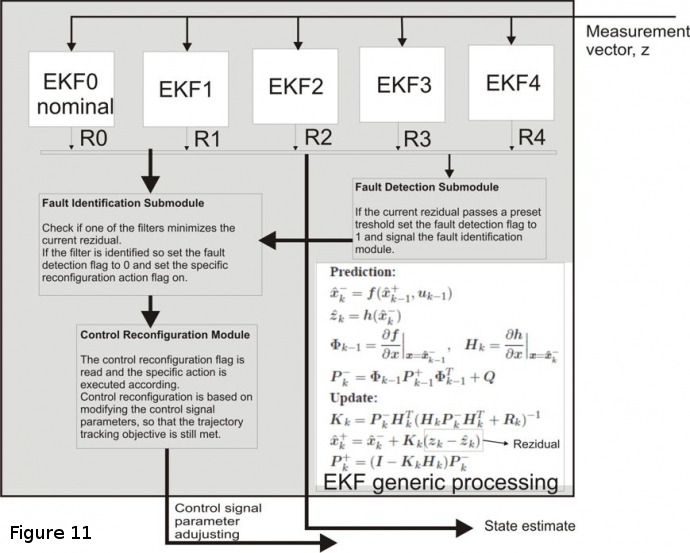
Competitions / Hackathons
Merck Research “Future of AI Challenge” (August 2019)
IRENA (Invariant Representations Extraction in Neural Architectures)
Team NeuroTHIx codebase. 1st Place at Merck Future of AI Research Challenge.
https://app.ekipa.de/challenge/future-of-ai/about
THI coverage:
https://www.thi.de/suche/news/news/thi-erfolgreich-in-ai-forschungswettbewerb
Merck Research Challenge aimed to generate insights from various disciplines that can lead to progress towards an understanding of invariant representation – that are novel and not based on Deep Learning.
IRENA (Invariant Representations Extraction in Neural Architectures) is the approach that team NeuroTHIx developed. IRENA offers a computational layer for extracting sensory relations for rich visual scenes, withy learning, inference, de-noising and sensor fusion capabilities. The system is also capable, through its underlying unsupervised learning capabilities, to embed semantics and perform scene understanding.
Using cortical maps as neural substrate for distributed representations of sensory streams, our system is able to learn its connectivity (i.e., structure) from the long-term evolution of sensory observations. This process mimics a typical development process where self-construction (connectivity learning), self-organization, and correlation extraction ensure a refined and stable representation and processing substrate. Following these principles, we propose a model based on Self-Organizing Maps (SOM) and Hebbian Learning (HL) as main ingredients for extracting underlying correlations in sensory data, the basis for subsequently extracting invariant representations.
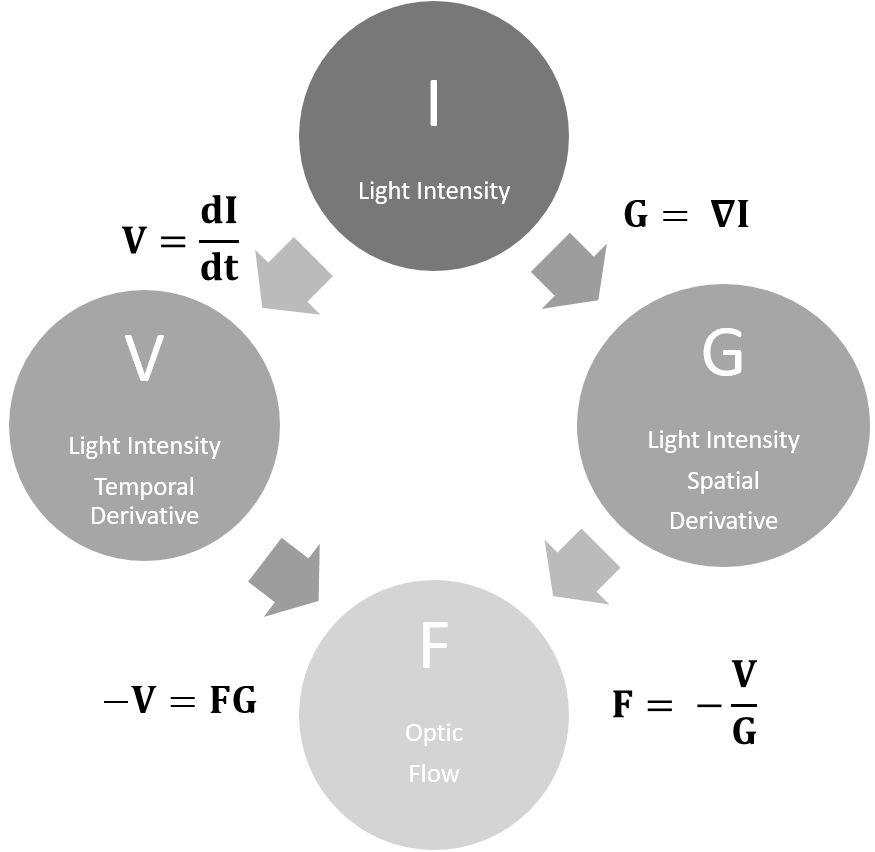
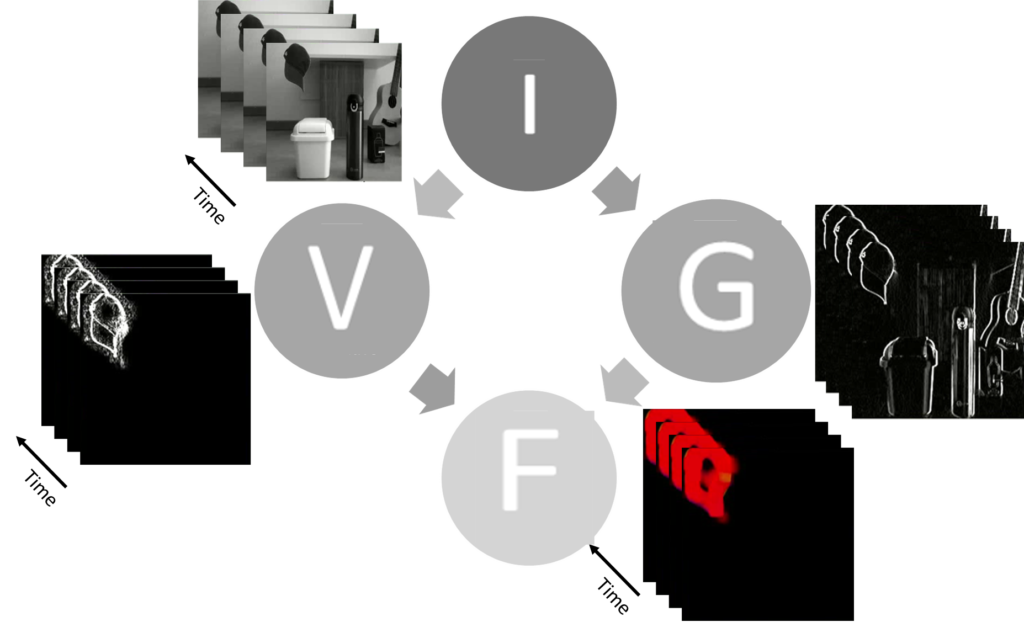
University of Cambridge Hackathon – Hack Cambridge (January 2017)
Microsoft Faculty Connection coverage (http://goo.gl/uPWGna) for project demo at Hack Cambridge 2017, 28 – 29 January 2017, University of Cambridge with a Real-time Event-based Vision Monitoring and Notification System for Seniors and Elderly using Neural Networks.
It has been estimated that 33% of people age 65 will fall. At around 80, that increases to 50%. In case of a fall, seniors who receive help within an hour have a better rate of survival and, the faster help arrives, the less likely an injury will lead to hospitalization or the need to move into a long-term care facility. In such cases fast visual detection of abnormal motion patterns is crucial.
In this project we propose the use of a novel embedded Dynamic Vision Sensor (eDVS) for the task of classifying falls. Opposite from standard cameras which provide a time sequenced stream of frames, the eDVS provides only relative changes in a scene, given by individual events at the pixel level. Using this different encoding scheme the eDVS brings advantages over standard cameras. First, there is no redundancy in the data received from the sensor, only changes are reported. Second, as only events are considered the eDVS data rate is high. Third, the power consumption of the overall system is small, as just a low-end microcontroller is used to fetch events from the sensor and can ultimately run for long time periods in a battery powered setup. This project investigates how can we exploit the eDVS fast response time and low-redundancy in making decisions about elderly motion.
The computation back-end will be realized with a neural network classification to detect fall and filter outliers. The data will be provided from 2 stimuli (blinking LEDs at different frequencies) and will represent the actual position of the person wearing them. The changes in position of the stimuli will encode the possible positions corresponding to falls or normal cases.
We will use Microsoft Azure ML Studio to implement a MLP binary classifier for the 4 (2 stimuli x 2 Cartesian coordinates – (x,y) in the field of view) dimensional input. We labelled the data with Fall (F) and No Fall (NF).
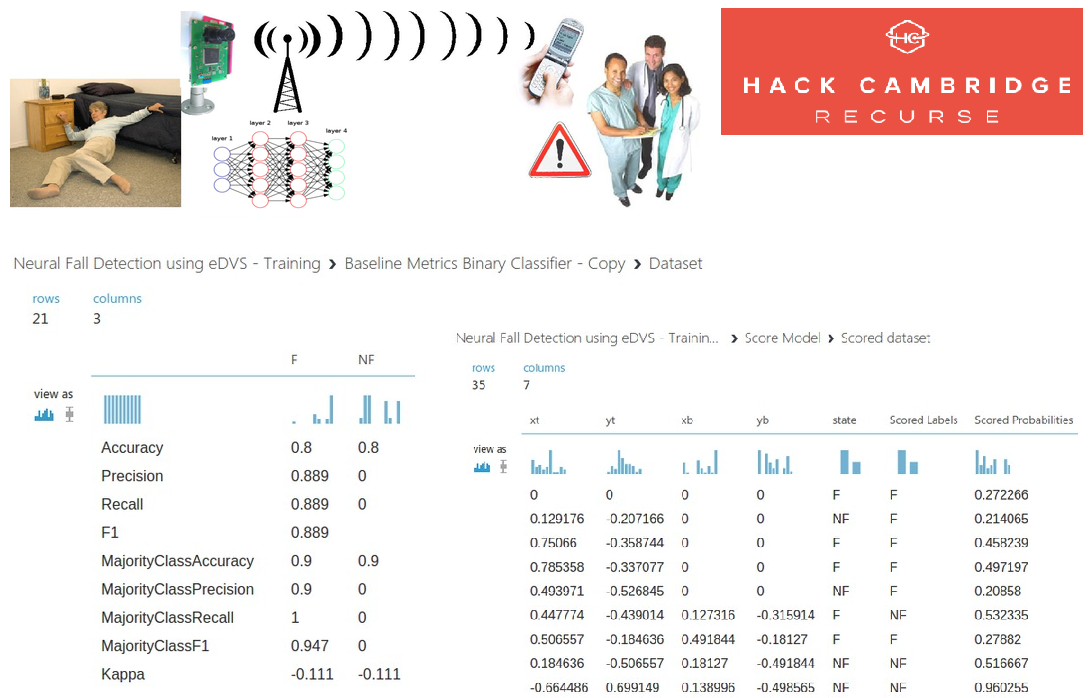
ANDRITZ Pioneers Hackathon (January 2017)
Best innovation idea at the ANDRITZ Pioneers Hackaton innovating for the international technology group ANDRITZ. Developed an artificial neural learning agent for automation process productivity enhancement.
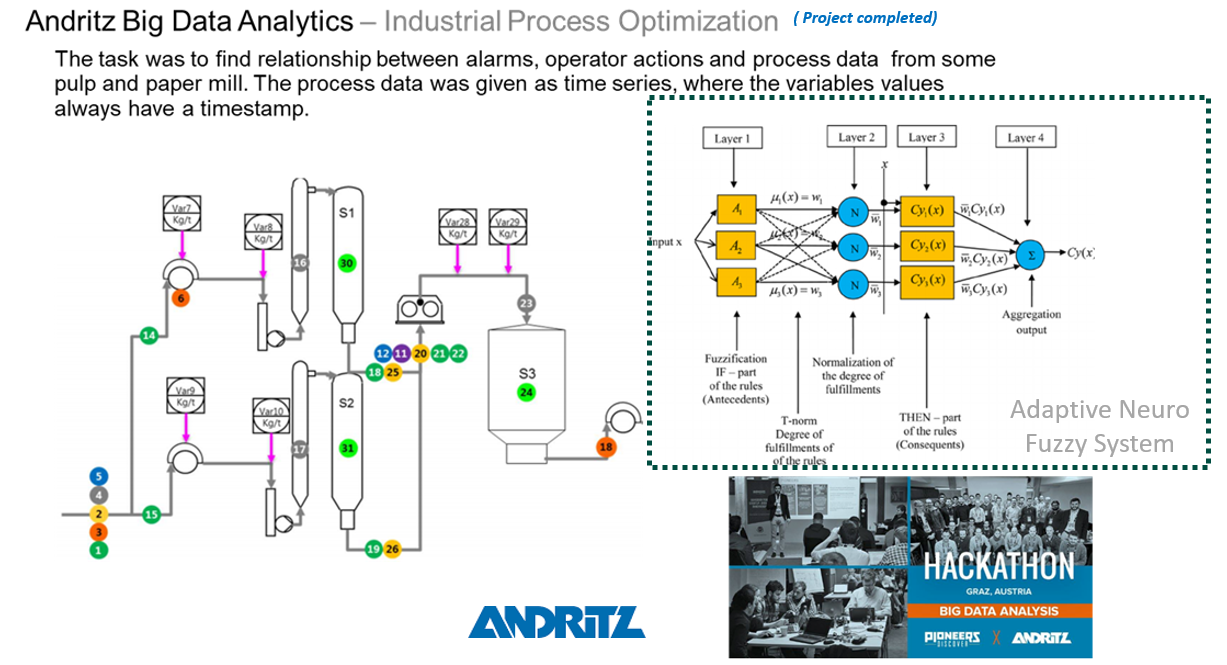
Wellcome Trust Hack The Senses Hackathon (June 2016)
WIRED UK coverage ( http://goo.gl/5yQ1Fn ) at the Hack the Senses hackathon in London: How to hack your senses: from ‘seeing’ sound to ‘hair GPS’: “Two-man team HearSee built a headband that taps into synaesthesia, translating changes in frame-less video to sound allowing blind people or those with a weak vision to see motion. Roboticist and neurologist Cristian Axenie assembled the hardware in mere minutes – attaching a pair of cameras and wires to a terrycloth headband.”
Daimler FinTech Hackathon (April 2016)
Awarded 1st prize (team) at the Daimler Financial Services Big Data Analytics Hackaton for the design of a neuro-fuzzy learning system for anomaly detection and user interaction in big data streams.
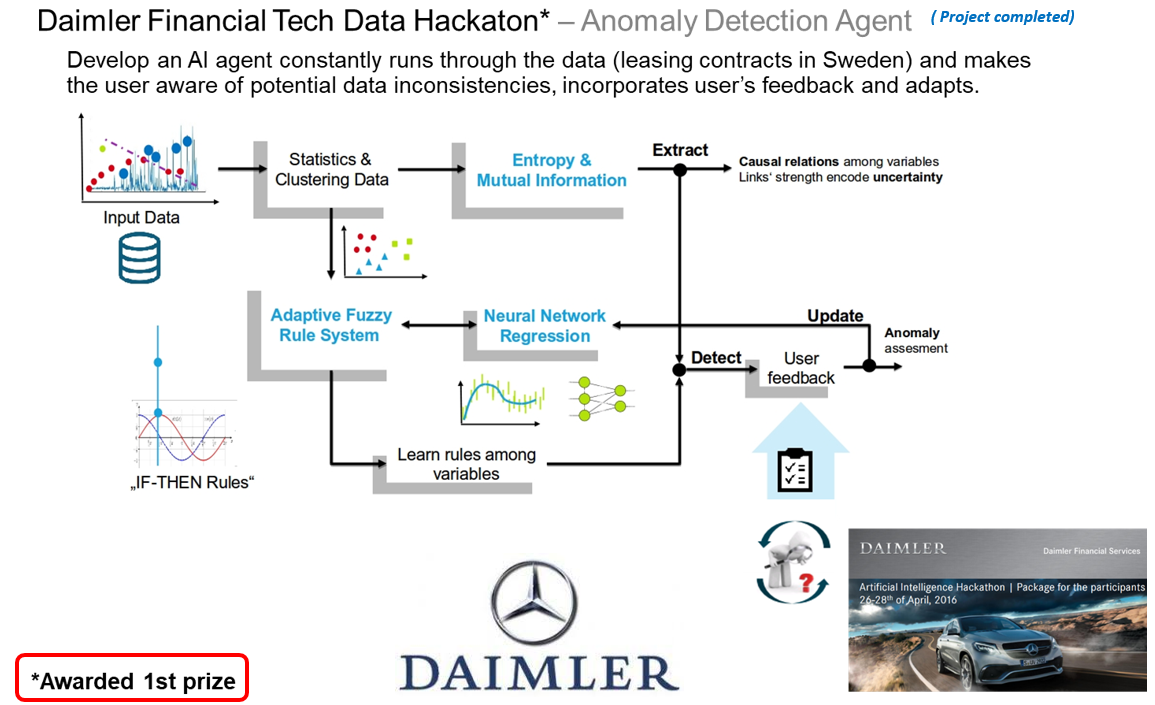
Burda Hackdays (April 2016)
Awarded special Microsoft Cognitive Technologies prize at the Burda Hackdays for the design of a neural learning system for inferring role assignments in working teams using psychometric data analytics.
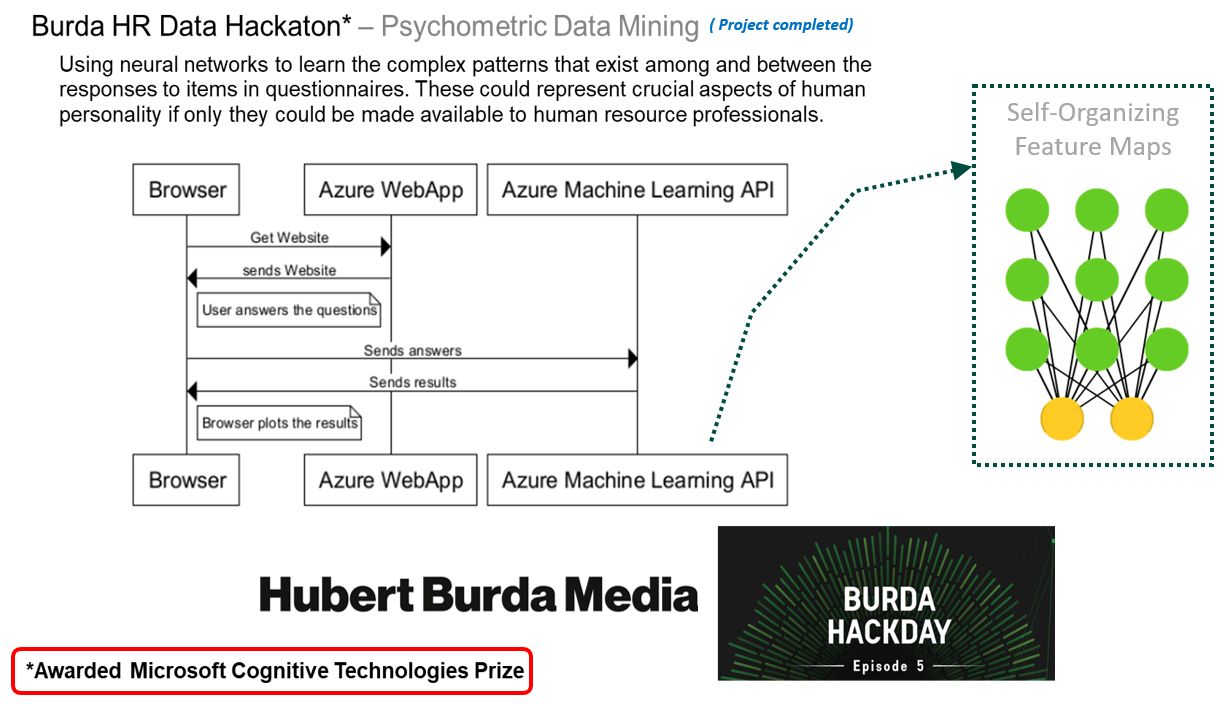
Automotive Hackdays (March 2016)
Awarded 1st prize (team) in the BMW Automotive Hackdays for the design of an inference system for driving profile learning and recommendation for skills improvement and predictive maintenance in car-sharing.
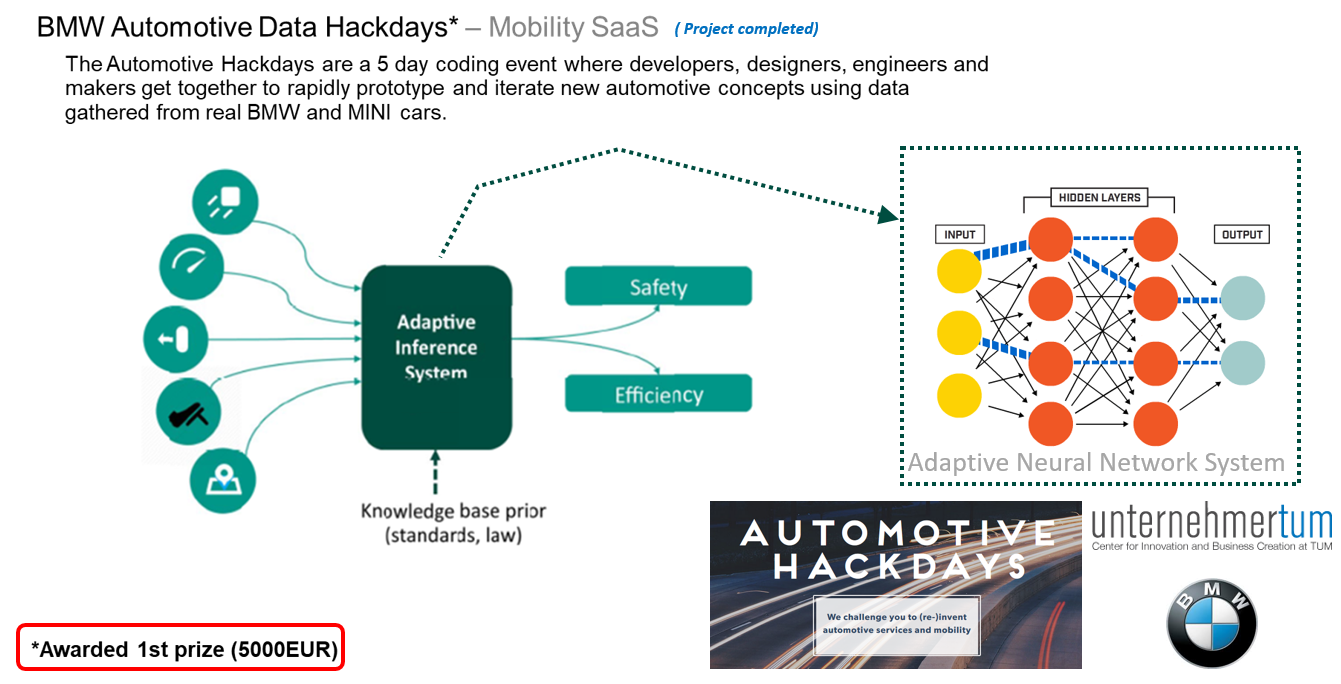