13. June 2020
Introduction
COMPONENS focuses on the research and development of tools, models and infrastructure needed to interpret large amounts of clinical data and enhance cancer treatments and our understanding of the disease. To this end, COMPONENS serves as a bridge between the data, the engineer, and the clinician in oncological practice.
Thus, knowledge-based predictive mathematical modelling is used to fill gaps in sparse data; assist and train machine learning algorithms; provide measurable interpretations of complex and heterogeneous clinical data sets, and make patient-tailored predictions of cancer progression and response.
GLUECK: Growth pattern Learning for Unsupervised Extraction of Cancer Kinetics
Neoplastic processes are described by complex and heterogeneous dynamics. The interaction of neoplastic cells with their environment describes tumor growth and is critical for the initiation of cancer invasion. Despite the large spectrum of tumor growth models, there is no clear guidance on how to choose the most appropriate model for a particular cancer and how this will impact its subsequent use in therapy planning. Such models need parametrization that is dependent on tumor biology and hardly generalize to other tumor types and their variability. Moreover, the datasets are small in size due to the limited or expensive measurement methods. Alleviating the limitations that incomplete biological descriptions, the diversity of tumor types, and the small size of the data bring to mechanistic models, we introduce Growth pattern Learning for Unsupervised Extraction of Cancer Kinetics (GLUECK) a novel, data-driven model based on a neural network capable of unsupervised learning of cancer growth curves. Employing mechanisms of competition, cooperation, and correlation in neural networks, GLUECK learns the temporal evolution of the input data along with the underlying distribution of the input space. We demonstrate the superior accuracy of GLUECK, against four typically used tumor growth models, in extracting growth curves from a set of four clinical tumor datasets. Our experiments show that, without any modification, GLUECK can learn the underlying growth curves being versatile between and within tumor types.
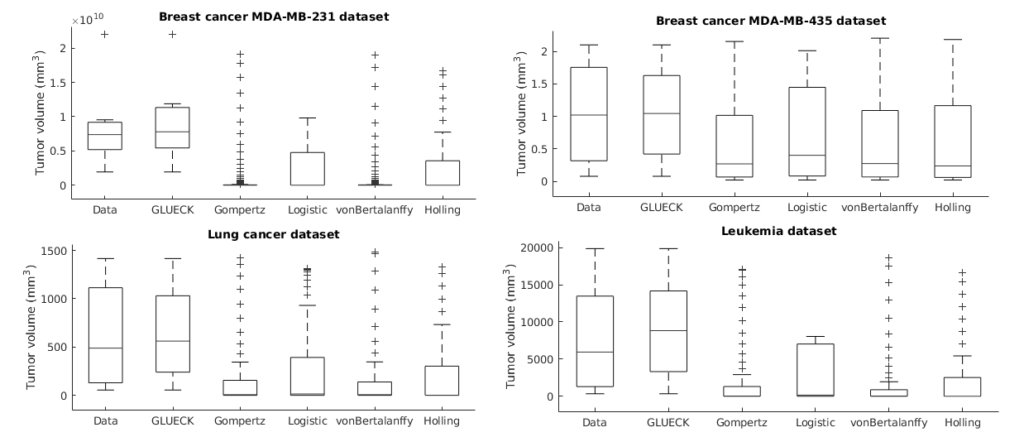
Preprint
https://www.biorxiv.org/content/10.1101/2020.06.13.140715v1
Code
https://gitlab.com/akii-microlab/ecml-2020-glueck-codebase
PRINCESS: Prediction of Individual Breast Cancer Evolution to Surgical Size
Modelling surgical size is not inherently meant to replicate the tumor’s exact form and proportions, but instead to elucidate the degree of the tissue volume that may be surgically removed in terms of improving patient survival and minimize the risk that a second or third operation will be needed to eliminate all malignant cells entirely. Given the broad range of models of tumor growth, there is no specific rule of thumb about how to select the most suitable model for a particular breast cancer type and whether that would influence its subsequent application in surgery planning. Typically, these models require tumor biology-dependent parametrization, which hardly generalizes to cope with tumor heterogeneity. In addition, the datasets are limited in size owing to the restricted or expensive methods of measurement. We address the shortcomings that incomplete biological specifications, the variety of tumor types and the limited size of the data bring to existing mechanistic tumor growth models and introduce a Machine Learning model for the PRediction of INdividual breast Cancer Evolution to Surgical Size (PRINCESS). This is a data-driven model based on neural networks capable of unsupervised learning of cancer growth curves. PRINCESS learns the temporal evolution of the tumor along with the underlying distribution of the measurement space. We demonstrate the superior accuracy of PRINCESS, against four typically used tumor growth models, in extracting tumor growth curves from a set of nine clinical breast cancer datasets. Our experiments show that, without any modification, PRINCESS can learn the underlying growth curves being versatile between breast cancer types.
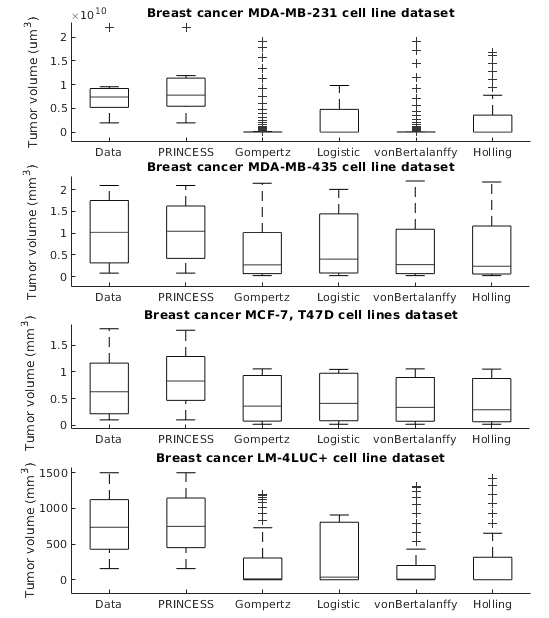
Preprint
https://www.biorxiv.org/content/10.1101/2020.06.13.150136v1
Code
https://gitlab.com/akii-microlab/cbms2020
TUCANN: TUmor Characterization using Artificial Neural Networks
Despite the variety of imaging, genetic and histopathological data used to assess tumors, there is still an unmet need for patient-specific tumor growth profile extraction and tumor volume prediction, for use in surgery planning. Models of tumor growth predict tumor size based on measurements made in histological images of individual patients’ tumors compared to diagnostic imaging. Typically, such models require tumor biology-dependent parametrization, which hardly generalizes to cope with tumor variability among patients. In addition, the histopathology specimens datasets are limited in size, owing to the restricted or single-time measurements. In this work, we address the shortcomings that incomplete biological specifications, the inter-patient variability of tumors, and the limited size of the data bring to mechanistic tumor growth models and introduce a machine learning model capable of characterizing a tumor, namely its growth pattern, phenotypical transitions, and volume. The model learns without supervision, from different types of breast cancer data the underlying mathematical relations describing tumor growth curves more accurate than three state-of-the-art models on three publicly available clinical breast cancer datasets, being versatile among breast cancer types. Moreover, the model can also, without modification, learn the mathematical relations among, for instance, histopathological and morphological parameters of the tumor and together with the growth curve capture the (phenotypical) growth transitions of the tumor from a small amount of data. Finally, given the tumor growth curve and its transitions, our model can learn the relation among tumor proliferation-to-apoptosis ratio, tumor radius, and tumor nutrient diffusion length to estimate tumor volume, which can be readily incorporated within current clinical practice, for surgery planning. We demonstrate the broad capabilities of our model through a series of experiments on publicly available clinical datasets.
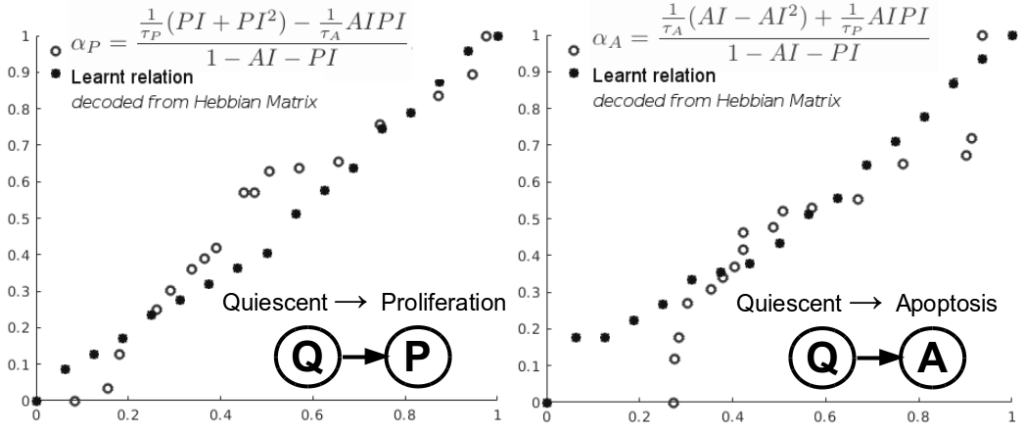
Preprint
https://www.biorxiv.org/content/10.1101/2020.06.08.140723v1
Code
https://gitlab.com/akii-microlab/icann-2020-bio
CHIMERA: Combining Mechanistic Models and Machine Learning for Personalized Chemotherapy and Surgery Sequencing in Breast Cancer
Mathematical and computational oncology has increased the pace of cancer research towards the advancement of personalized therapy. Serving the pressing need to exploit the large amounts of currently underutilized data, such approaches bring a significant clinical advantage in tailoring the therapy. CHIMERA is a novel system that combines mechanistic modelling and machine learning for personalized chemotherapy and surgery sequencing in breast cancer. It optimizes decision-making in personalized breast cancer therapy by connecting tumor growth behaviour and chemotherapy effects through predictive modelling and learning. We demonstrate the capabilities of CHIMERA in learning simultaneously the tumor growth patterns, across several types of breast cancer, and the pharmacokinetics of a typical breast cancer chemotoxic drug. The learnt functions are subsequently used to predict how to sequence the intervention. We demonstrate the versatility of CHIMERA in learning from tumor growth and pharmacokinetics data to provide robust predictions under two, typically used, chemotherapy protocol hypotheses.
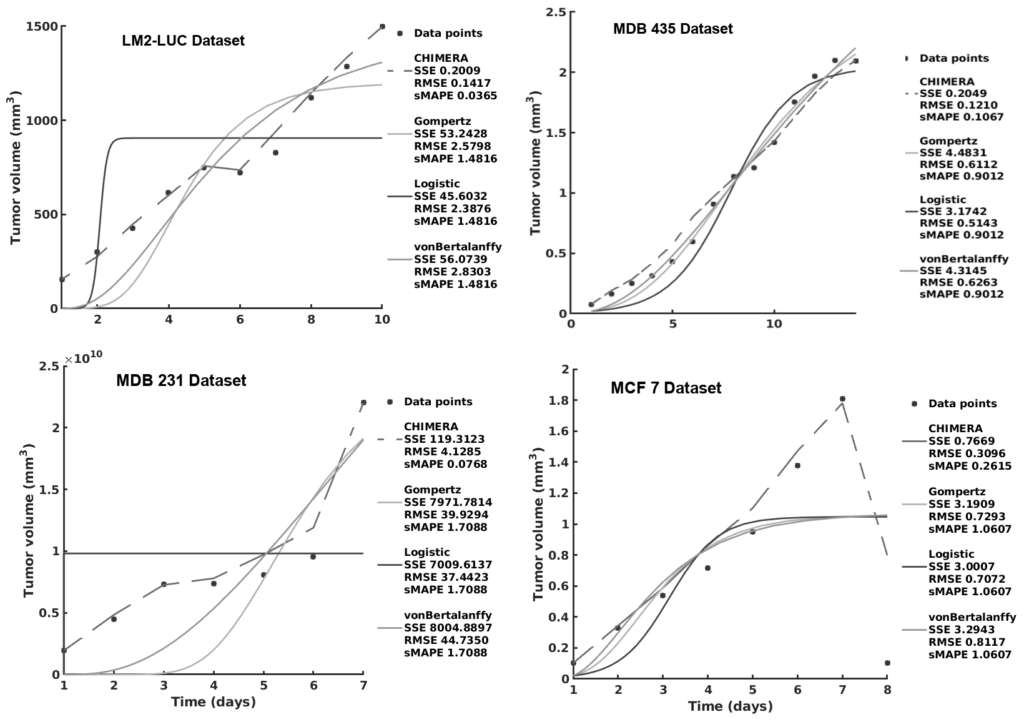
Preprint
https://www.biorxiv.org/content/10.1101/2020.06.08.140756v1
Code
https://gitlab.com/akii-microlab/bibe2020